Introduction: Understanding the Essence
In the dynamic world of data analysis, uncovering the hidden narratives within your data is a superpower. Adeptly navigating meaningful relationships between variables allows you to decode behaviors, optimize strategies, and make decisions that resonate with your target audience. These relationships empower you to discern patterns, predict outcomes, and fine-tune your approaches for maximum impact.
Why It Matters
Understanding the intricate web of relationships between variables is pivotal. It’s the compass guiding the way through the vast landscape of preferences. This skill enables you to optimize campaigns, ensuring that every targeted effort aligns seamlessly with your audience. By examining the connections between different factors, you can craft strategies that resonate, predict trends, and ultimately drive success.
The Practical Importance
The practical significance lies in the actionable insights that emerge from understanding relationships. Imagine predicting how changes in product quality influence customer satisfaction or understanding how customer age correlates with responses to seasonal promotions. These insights allow you to tailor your approaches, refine targeting, and enhance overall effectiveness.
The Guide: A Step-by-Step Approach
Let’s walk through the practical steps to unlock these meaningful relationships:
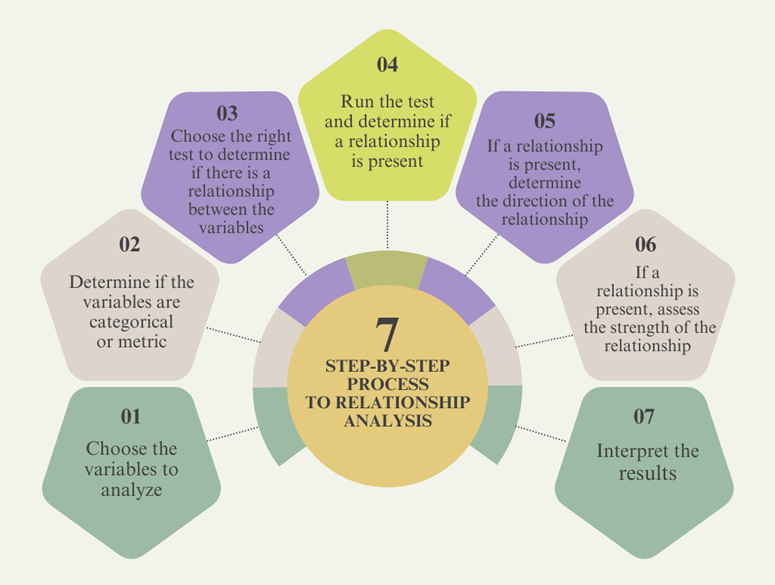
Step 1: Choose the Variables to Analyze
At the outset, identify the variables that matter. For instance, you might choose to understand the relationship between social media engagement and product purchases.
Step 2: Determine Variable Type
Classify your chosen variables as categorical or metric. If exploring customer satisfaction ratings (metric) and product categories (categorical), this step provides clarity for subsequent analyses.
Step 3: Choose the Right Test
Select the appropriate test based on variable type. Suppose you want to confirm the relationship between customer feedback (categorical) and overall satisfaction scores (metric). Employ a chi-square analysis and correlation coefficient to reveal the nuances.
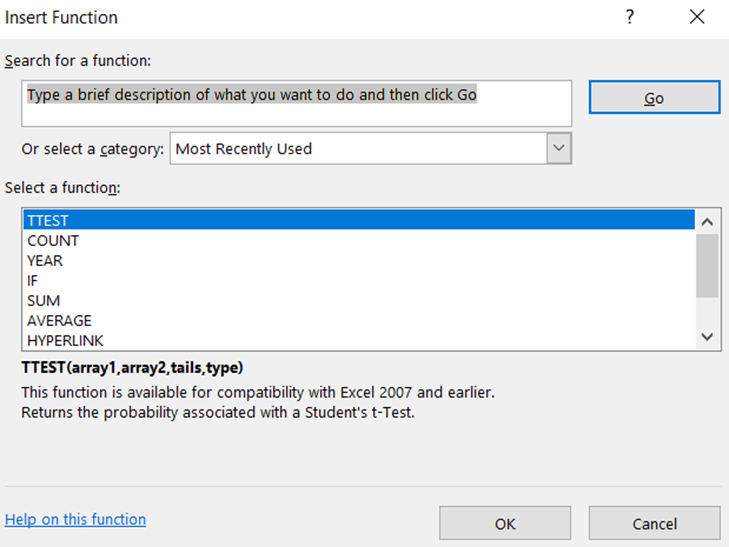
Step 4: Run the Test
Practical execution involves using tools like Excel to process raw data. Through this step, you transform numbers into meaningful insights, visualizing how changes in one variable impact another.
Step 5: Assess Relationship Presence
Analyzing the p-value now carries deeper significance. When the p-value falls below the predetermined significance level, it empowers you to assert with confidence the existence of a relationship. This not only fortifies your strategies but also imbues them with statistical significance, providing a robust foundation for data-driven decision-making.
Step 6: Evaluate Relationship Strength
In this stage, evaluate the robustness of the identified relationship. Analyze the correlation coefficient or other relevant metrics to gauge the intensity of the connection between variables. This step provides insights into how influential one variable is on another, aiding in the overall comprehension of the relationship’s strength.
Step 7: Interpret the Results
Now that the tests are complete, it’s time to delve into the results. Analyze the data patterns, assess any discovered relationships, and draw insightful conclusions. This crucial step involves translating statistical outcomes into meaningful insights that can inform strategic decisions.
Conclusion: Empowering You with Insights
Mastering the analysis of meaningful relationships isn’t just theoretical; it’s about transforming data into strategies. This guide equips you with both the theory and practical skills to extract actionable insights. Stay tuned for accompanying tutorials, ensuring you seamlessly integrate these insights into your strategies for impactful results.